Are you ready to implement AI in your business but need help figuring out where to start? AI implementation can streamline operations and catalyze growth, yet requires a thoughtful approach. This guide cuts through the complexity, offering practical steps to align AI with your strategic goals, navigate technology selection, and establish a robust data foundation. Tailored for decision-makers, it also sheds light on building the right team and integrating AI into business processes without disruption.
Fundamental Insights on AI Implementation
- The successful implementation of AI in business begins with setting dynamic AI-driven objectives and goals that align with strategic business outcomes, focusing on high-impact areas and continuous updating.
- Choosing the optimal AI solution requires considering factors such as compatibility with existing systems, scalability, flexibility, and whether to use ready-made services or custom solutions, alongside building a robust data strategy and a skilled AI team.
- Integrating AI into business operations involves a phased approach, minimizing disruption, and providing employee training, while monitoring and evaluating AI performance is essential using KPIs aligned with business goals, constantly looking for enhancement opportunities, and addressing ethical and legal considerations.
Establishing AI-Driven Objectives
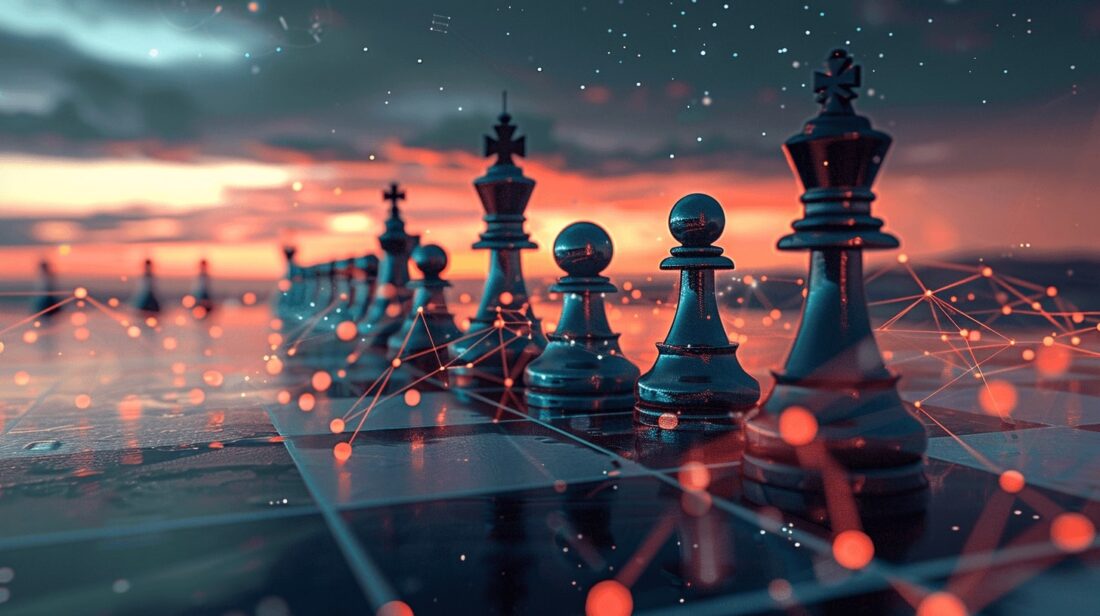
Embarking on the exciting journey of successful AI implementation in your organization begins with setting clear and concrete goals. It’s important to understand that AI isn’t an instant solution for transforming business operations but a potent instrument that, if properly aligned with strategic objectives, can drive substantial improvements in operational efficiency and productivity.
It is critical to ensure that the goals for your AI initiatives are not static. They should evolve as technology advances and your business’s needs change. Continually revising and updating dynamic objectives allows you to remain at the forefront of innovation and keep a competitive advantage in your industry.
Identifying Key Areas for AI Integration
Incorporating AI will not uniformly impact every aspect of business. Specifically, areas such as customer service, marketing, and operations are on the cusp of radical changes that appropriate AI solutions could significantly augment. Concentrating efforts in these pivotal sectors can amplify the rewards gleaned from your AI projects while ensuring that resources are allocated efficiently.
It’s crucial to recognize that successful integration of AI depends heavily on how well it fits with your existing technological framework and the skill set of your workforce. Carefully pinpointing prime scenarios for applying AI within your organization and evaluating their potential benefits and practicability is essential for gaining a strategic edge.
Assessing Business Needs and Opportunities
Embarking on the path to integrating AI should involve:
- Assessing how AI might influence your business operations, enhance income generation, and slash expenses
- Executing a thorough cost-benefit assessment to rank AI initiatives by their prospective investment returns
- Detecting areas of resistance within customer experiences and internal workflows to direct strategic uses of AI
- Rigorously filtering opportunities down to those that can be feasibly applied
Remember that the success of implementing AI is deeply connected with its congruence with your broad business objectives and aims.
Selecting the Optimal AI Solution

Once you have established an AI implementation strategy with clear AI goals and identified the primary areas for integration, your next step is to select the most suitable AI solution for your business. This choice should take into account a balance of human resources, operational processes, and technological needs with an emphasis on:
- scalability
- flexibility
- ease of integration
- compatibility with current systems
The chosen AI solution must be in sync with user necessities and their level of technical knowledge to ensure that its features and functions correspond to what users require.
You may choose between adopting off-the-shelf AI services for their time-saving nature and cost-effectiveness or customizing an AI solution designed specifically to meet unique requirements. Remember that external expertise can be vital in expediting your organization’s development and assimilation of prospective AI solutions.
Evaluating AI Technologies
Artificial intelligence encompasses various technologies, each tailored for specific uses. Machine learning is fundamental to customer verification (KYC), identifying fraudulent activities, and upholding regulatory compliance. At the same time, natural language processing underpins the operation of conversational agents and emotional tone analysis.
When selecting an AI system that fits best with your operations:
- Assess the existing skill set within your organization
- Examine how components are integrated into AI systems—whether they’re based in the cloud, on-edge devices, through a hybrid model, or via distributed frameworks.
- Decide if pre-built AI solutions suit your requirements more effectively than custom-developed ones to align closely with your resources and goals.
Analyzing Compatibility with Existing Systems
AI solutions should be regarded not as isolated entities but as vital components that blend into the extensive technological frameworks of your business model. To guarantee the AI solution meshes well and functions without disruption in your existing setup, it is imperative to thoroughly examine your current technical infrastructure, data structures, and communication standards.
Tackling obstacles like segregated data repositories and interconnection hurdles is pivotal for cultivating a data habitat conducive to the adoption of AI. Adhering to established protocols for fusing AI solutions with age-old systems will facilitate an effortless shift towards AI-enhanced workflows.
Crafting a Robust Data Strategy

Raw data stands as the foundational element of artificial intelligence. A robust strategy for this data is imperative. Without it, AI endeavors are at risk of failure. An effective strategy outlines leveraging data and pinpoints specific use cases that satisfy business requirements, ranging from traditional analytics to cutting-edge AI initiatives. Breaking down organizational barriers and advocating for data integration are critical steps in formulating a comprehensive asset and data management approach. Remember that proper governance is vital; it ensures accuracy in your datasets while preventing errors and privacy breaches.
It’s important to remember that if you input poor-quality raw data into your systems, poor outputs will ensue—as reflected by the principle “garbage in, garbage out.” The integrity of an AI system’s output directly reflects its initial raw dataset quality.
Data Acquisition and Management
The initial phase of crafting an effective AI model hinges on the data acquisition process. It requires gathering, purifying, and structuring data to be readily available and easily handled. Establishing stringent data governance is vital in maintaining the integrity of the data, safeguarding against mistakes, and protecting privacy.
It should be noted that AI models thrive in a setting where there’s diligent management and oversight of data quality, detailed attention to metadata handling, and protected access to varied training datasets. The responsibility falls heavily on data engineers to develop this type of infrastructure.
Leveraging Analytics for Decision-Making
In today’s Big Data era, processing vast amounts of information for strategic decision-making is a critical edge in the competitive landscape. With AI technology, data scientists offer analytics that yield valuable insights that are instrumental in steering business strategies. Such insights enable organizations to:
- Remain proactive and ahead of industry changes
- Detect emerging trends and discern patterns
- Enhance operational efficiencies
- Elevate customer experiences
- Foster innovation
Employing a centralized repository to store data and disseminate these findings ensures stakeholders can swiftly make well-informed choices. It’s important to note that an orderly arrangement of data topologies aids in managing various data situations, which is essential for keeping data frameworks and AI technology up-to-date—thus improving analytical capabilities significantly.
Assembling a Skilled AI Team

AI, as a multifaceted domain, necessitates an array of capabilities. For AI projects to thrive, the team must possess expertise in:
- Software engineering
- Data Analytics
- Machine learning techniques
- Domain-specific knowledge
Having a machine learning team with varied perspectives enables the posing of distinct questions, which aids in spotting and curbing possible biases within AI models. Combining machine learning engineers from such a diverse team marks only the initial phase.
It’s equally important to foster creativity among members of the AI group, pinpoint competent leaders who can steer efforts effectively, and orchestrate management strategies for various AI initiatives, ensuring their successful outcomes.
Internal vs. External Talent
Deciding whether to use in-house staff or outsource AI expertise is a significant choice that affects the outcome of your AI projects. Opting for internal staff can lead to a more profound commitment to the company’s objectives, while outsourcing allows for instant cost savings since there are no enduring wage obligations. You may either enhance your existing employees’ skills through training programs or capitalize on the specialized abilities and new insights outside AI professionals provide. Each path has its unique benefits and potential pitfalls.
Fostering a Data-Driven Culture
For AI to be effectively incorporated, establishing a data-driven culture is crucial. This requires advancing data literacy, openness, and an experimental mindset that fosters the embrace of AI and its innovative application. To deeply instill a culture centered on data within a company, it’s beneficial to successfully implement AI, training initiatives, and tailored development strategies.
Embedding data analytics into everyday business processes while progressively expanding AI skills can increase the organization’s confidence in these technologies. Such steps are essential for promoting wider acceptance and integration of AI across various functions.
Training and Fine-Tuning AI Models

Educating an AI model is recursive and necessitates a vast, all-encompassing training data set. This process includes executing trials or simulations, forecasting outcomes, and refining the model over various training rounds to improve precision. The enhancement in the accuracy stems from assessing predicted outcomes against actual training data and implementing essential adjustments to perfect the AI model.
Remember that triumphantly administering AI initiatives within a corporation hinges on surmounting any reluctance towards change.
Model Development and Validation
Creating an AI model entails selecting the optimal machine learning approach tailored to the given application through reinforcement learning or deep learning techniques. Such models must be thoroughly validated with appropriate datasets to maintain their performance. This step is crucial within the framework of ML governance, which encompasses managing access controls, enacting policies, and monitoring activities associated with the model.
Establishing KPIs for AI—like Mean Squared Error (MSE)—plays a vital role in assessing how effectively machines perform tasks and allows for early detection and management of outlier effects.
Continuous Improvement and Adaptation
AI systems must undergo continual training and updates in the rapidly advancing domain of artificial intelligence. This ensures they stay relevant as data patterns shift and business contexts change. Embracing a step-by-step methodology in executing AI projects allows these systems to benefit from periodic enhancements, elevating their precision while diminishing biases through consistent feedback integration.
Transfer learning offers an efficient strategy for tailoring pre-existing AI models to novel situations. This technique refines the accuracy and relevance of models by adapting them specifically to new tasks without starting from scratch each time.
Integrating AI into Business Operations
Successfully implementing AI is measured by how effortlessly it becomes a part of existing business operations. Ensuring that the infrastructure is ready for the implementation of AI is essential to efficiently train, maintain, and deploy AI algorithms within a regulated setting. This foundational work aids in managing any unpredictability once these systems are operational in actual business environments.
It’s imperative to limit disturbances throughout the integration process of AI to guarantee uninterrupted business activities, thereby averting any cessation or decline in productivity.
Minimizing Disruption
When introducing new technologies, the risk of disruption is always present. By employing a phased approach to deployment, AI systems can be integrated into distinct areas of business in stages to reduce disturbances. Implementing a parallel run strategy enables these AI systems to work alongside current legacy systems for a time, ensuring an easier shift.It’s essential to remember this and ensure that AI systems undergo extensive testing within controlled environments before deploying them extensively. This is key for limiting any interruptions they might cause to ongoing processes.
Employee Training and Support
Transitioning to AI-powered operations demands a technological shift and a significant human adaptation. Tailoring AI training programs to cater to the distinct roles of employees can boost their self-assurance when engaging with processes that AI drives.
Maintaining continuous assistance for personnel through helpdesks or specialized teams dedicated to AI support is crucial. This is essential in aiding staff members as they navigate any obstacles encountered while adapting both new tools and workflows involving AI.
Monitoring and Evaluating AI Performance
After your AI systems become operational, tracking and assessing their performance is crucial. To do this effectively, you should use key performance indicators (KPIs) that capture not only quantitative metrics like:
- mean time to repair (MTTR)
- accuracy rate
- response time
- throughput
However, qualitative factors should also be included to ensure a comprehensive evaluation of the system’s success and highlight opportunities for advancement.
Bear in mind that employing advanced analytics can be instrumental in:
- Keeping tabs on and evaluating the effectiveness of AI deployments.
- Helping organizations quickly identify problems.
- Refining processes.
- Modifying tactics to achieve better outcomes.
Aligning KPIs with Business Goals
Ensuring that the performance metrics for AI are in harmony with the broader objectives of a business is paramount. Defining success in AI must be done within the context of an organization’s aims, selecting key performance indicators (KPIs) that mirror impacts on the business—be it cost reduction, heightened efficiency, or bolstered customer satisfaction.
It is important to note that a data strategy can only be deemed successful if it’s connected to prioritized business outcomes. Such a business strategy also employs quantifiable metrics to steer and measure AI initiatives and acknowledges the critical role of customer data.
Identifying Opportunities for Enhancement
Performance assessment goes beyond merely determining the efficacy of your AI systems. It encompasses recognizing prospects for advancement. By employing Key Performance Indicators such as Mean Time to Repair (MTTR) and customer satisfaction, companies can pinpoint aspects needing refinement within technical performance and the overall consumer experience.
Remember that harnessing AI to create customized improvement plans and instant feedback is vital when seeking opportunities to bolster performance during evaluations.
Addressing Ethical and Legal Considerations
The increasing ubiquity of AI brings ethical and legal issues into sharper focus. To ensure responsible deployment, the clarity of AI processes is essential for fostering accountability and building trust, which are fundamental in addressing AI’s ethical and legal challenges.
It’s important to note that adhering to regulations specific to each industry is vital because failing can result in substantial financial fines and severe damage to a company’s reputation.
Mitigating Bias and Ensuring Fairness
Addressing bias in AI models is a critical issue that must be dealt with during the training of AI models. By thoroughly understanding and taking responsibility for the training data, one can minimize unexpected biases arising from various classes and labels within datasets.
It’s essential to remember that when you are engaged in training an AI model, you should actively work on removing any elements within your data that have the potential to distort the model’s forecasts or lead to discriminatory outcomes.
Compliance with Industry-Specific Regulations
Abiding by regulations specific to an industry is critical not merely to evade fines but equally for preserving credibility and reputation. Enterprises must synchronize their Artificial Intelligence (AI) and Machine Learning (ML) protocols with regulatory standards pertinent to their sector, encompassing aspects such as:
- The protection of data privacy
- Ensuring robust security measures
- Meticulous record management
- Conforming to auditing stipulations
It’s important to remember that adherence to these specialized regulations is imperative. Failing organizations may face substantial economic sanctions and severe damage to their reputation.
Summary
As we’ve explored the essential steps towards harnessing the transformative power of AI in your business, from setting dynamic objectives to navigating technological selections and fostering a data-driven culture, it’s clear that the journey requires expertise, strategic planning, and continuous innovation. If you find yourself ready to embark on this transformative journey but need guidance or support, Koombea stands prepared to assist. With our deep expertise in AI implementation and a track record of driving digital transformation, we can help streamline your AI integration process, ensuring your business reaps the full benefits of AI technologies. Explore AI Development Services with Koombea as your partner in navigating the complexities of AI implementation, enabling you to unlock new efficiencies, innovations, and growth opportunities. Contact us today to discover how our AI solutions can align perfectly with your business goals.
Frequently Asked Questions
What is AI implementation?
The integration of artificial intelligence within business operations is designed to streamline and enhance processes through automation, assist in making informed decisions, and accomplish clearly defined objectives that propel business performance. This process entails identifying particular tasks for AI applications, gathering and analyzing pertinent data, choosing suitable AI models, and incorporating them seamlessly into existing systems.
What are the stages of AI implementation?
Implementing an AI solution encompasses several critical stages, such as gathering and analyzing data, purifying it, exploring its intricacies, creating models, evaluating and interpreting these models’ performance, and the production deployment of the system, followed by ongoing maintenance. These steps require the collaboration of diverse specialists to engineer a proficient AI solution.
How do you implement an AI project?
When embarking on an AI project, a six-step methodology aids in catering to the business needs and tackling challenges associated with such projects. This strategy includes grasping the commercial context, a robust data pipeline, comprehending the data, readying it for use, developing models, assessing their performance, and deploying them into production.
What areas of a business can benefit most from AI integration?
Incorporating AI into critical sectors of business value, such as customer support, advertising, and business processes, can significantly enhance a company.
What significant concern needs to be addressed during AI model training?
It is essential to tackle prejudice within AI models throughout the AI implementation training phase to guarantee equitable and precise outcomes in their decision-making capabilities.